Apr 14, 2018 Implement all the functions of a dictionary (ADT) using hashing. Data: Set of (key, value) pairs, Keys are mapped to values, Keys must be comparable, Keys must be unique Standard Operations: Insert(key, value), Find(key), Delete(key). But these hashing function may lead to collision that is two or more keys are mapped to same value. Chain hashing avoids collision. The idea is to make each cell of hash table point to a linked list of records that have same hash function value. C program for hashing with chaining.
Hash TablesBy Eric SuhHash tables are an efficient implementation of a keyed array data structure,a structure sometimes known as an associative array or map. If you're workingin C, you can take advantage of thefor keyed arrays implemented using, butthis article will give you some of the theory behind how a hash tableworks.
Keyed Arrays vs. Indexed ArraysOne of the biggest drawbacks to a language like C is that there are nokeyed arrays. In a normal C array (also called an indexed array), the only wayto access an element would be through its index number. To find element 50 ofan array named 'employees' you have to access it like this:employees50;In a keyed array, however, you would be able to associate each element with a 'key,' which can be anything from a name to a product model number. So, if you have a keyed array of employee records, you could access the record of employee 'John Brown' like this:employees'Brown, John';One basic form of a keyed array is called the hash table.
In a hash table, akey is used to find an element instead of an index number. Since the hash tablehas to be coded using an indexed array, there has to be some way oftransforming a key to an index number. That way is called the hashing function. Hashing FunctionsA hashing function can be just about anything. How the hashing function isactually coded depends on the situation, but generally the hashing functionshould return a value based on a key and the size of the array the hashingtable is built on. Also, one important thing that is sometimes overlooked isthat a hashing function has to return the same value every time it is given thesame key.Let's say you wanted to organize a list of about 200 addresses by people'slast names.
A hash table would be ideal for this sort of thing, so that you canaccess the records with the people's last names as the keys.First, we have to determine the size of the array we're using. Let's use a 260 element array so that there can be an average of about 10 element spaces per letter of the alphabet.Now, we have to make a hashing function. First, let's create a relationship between letters and numbers:A - 0B - 1C - 2D - 3.and so on until Z - 25.The easiest way to organize the hash table would be based on the first letter of the last name.Since we have 260 elements, we can multiply the first letter of the last name by 10. So, when a key like 'Smith' is given, the key would be transformed to the index 180 (S is the 19 letter of the alphabet, so S - 18, and 18. 10 = 180).Since we use a simple function to generate an index number quickly, and we use the fact that the index number can be used to access an element directly, a hash table's access time is quite small. A of keys and elements wouldn't be nearly as fast, since you would have to search through every single key-element pair.
Collisions and Collision HandlingProblems, of course, arise when we have last names with the same first letter. So 'Webster' and 'Whitney' would correspond to the same index number, 22. A situation like this when two keys get sent to the same location in the array is called a collision. If you're trying to insert an element, you might find that the space is already filled by a different one.Of course, you might try to just make a huge array and thus make it almost impossible for collisions to happen, but then that defeats the purpose of using a hash table.
One of the advantages of the hash table is that it is both fast and small. Collision handling with open addressingThe simplest collision handling algorithm is known as the open address method or the closed hashing method. When you are adding an element, say 'Whitney,' and you find that another element is already there ('Webster,' for instance) then you would just proceed to the next element space (the one after 'Webster'). If that is filled, you go on to the next one, and so on, until you find an empty space to insert the new element (all those extra elements came in handy after all!).220 'White'.
HashingIn previous sections we were able to make improvements in our searchalgorithms by taking advantage of information about where items arestored in the collection with respect to one another. For example, byknowing that a list was ordered, we could search in logarithmic timeusing a binary search. In this section we will attempt to go one stepfurther by building a data structure that can be searched in(O(1)) time. This concept is referred to as hashing.In order to do this, we will need to know even more about where theitems might be when we go to look for them in the collection.
If everyitem is where it should be, then the search can use a single comparisonto discover the presence of an item. We will see, however, that this istypically not the case.A hash table is a collection of items which are stored in such a wayas to make it easy to find them later. Each position of the hash table,often called a slot, can hold an item and is named by an integervalue starting at 0. For example, we will have a slot named 0, a slotnamed 1, a slot named 2, and so on.
Initially, the hash table containsno items so every slot is empty. We can implement a hash table by usinga list with each element initialized to the special Python valueNone. Shows a hash table of size (m=11).In other words, there are m slots in the table, named 0 through 10. Figure 5: Hash Table with Six ItemsNow when we want to search for an item, we simply use the hash functionto compute the slot name for the item and then check the hash table tosee if it is present. This searching operation is (O(1)), sincea constant amount of time is required to compute the hash value and thenindex the hash table at that location.
If everything is where it shouldbe, we have found a constant time search algorithm.You can probably already see that this technique is going to work onlyif each item maps to a unique location in the hash table. For example,if the item 44 had been the next item in our collection, it would have ahash value of 0 ( (44% 11 0)). Since 77 also had a hashvalue of 0, we would have a problem.
According to the hash function, twoor more items would need to be in the same slot. This is referred to asa collision (it may also be called a “clash”). Clearly, collisionscreate a problem for the hashing technique. We will discuss them indetail later. Figure 7: Hashing a String Using Ordinal Values with WeightingYou may be able to think of a number of additional ways to compute hashvalues for items in a collection.
The important thing to remember isthat the hash function has to be efficient so that it does not becomethe dominant part of the storage and search process. If the hashfunction is too complex, then it becomes more work to compute the slotname than it would be to simply do a basic sequential or binary searchas described earlier. This would quickly defeat the purpose of hashing.
Collision ResolutionWe now return to the problem of collisions. When two items hash to thesame slot, we must have a systematic method for placing the second itemin the hash table. This process is called collision resolution.
Aswe stated earlier, if the hash function is perfect, collisions willnever occur. However, since this is often not possible, collisionresolution becomes a very important part of hashing.One method for resolving collisions looks into the hash table and triesto find another open slot to hold the item that caused the collision. Asimple way to do this is to start at the original hash value positionand then move in a sequential manner through the slots until weencounter the first slot that is empty.
Note that we may need to go backto the first slot (circularly) to cover the entire hash table. Thiscollision resolution process is referred to as open addressing inthat it tries to find the next open slot or address in the hash table.By systematically visiting each slot one at a time, we are performing anopen addressing technique called linear probing.shows an extended set of integer items under thesimple remainder method hash function (54,26,93,17,77,31,44,55,20).above shows the hash values for the original items.shows the original contents. When we attempt toplace 44 into slot 0, a collision occurs. Under linear probing, we looksequentially, slot by slot, until we find an open position. In thiscase, we find slot 1.Again, 55 should go in slot 0 but must be placed in slot 2 since it isthe next open position.
The final value of 20 hashes to slot 9. Sinceslot 9 is full, we begin to do linear probing. We visit slots 10, 0, 1,and 2, and finally find an empty slot at position 3. Figure 8: Collision Resolution with Linear ProbingOnce we have built a hash table using open addressing and linearprobing, it is essential that we utilize the same methods to search foritems. Assume we want to look up the item 93. When we compute the hashvalue, we get 5. Looking in slot 5 reveals 93, and we can returnTrue.
C Program To Implement Dictionary Using Hashing Functions In Excel
What if we are looking for 20? Now the hash value is 9, andslot 9 is currently holding 31. We cannot simply return False sincewe know that there could have been collisions. We are now forced to do asequential search, starting at position 10, looking until either we findthe item 20 or we find an empty slot.A disadvantage to linear probing is the tendency for clustering;items become clustered in the table.
This means that if many collisionsoccur at the same hash value, a number of surrounding slots will befilled by the linear probing resolution. This will have an impact onother items that are being inserted, as we saw when we tried to add theitem 20 above. A cluster of values hashing to 0 had to be skipped tofinally find an open position. This cluster is shown in. Figure 9: A Cluster of Items for Slot 0One way to deal with clustering is to extend the linear probingtechnique so that instead of looking sequentially for the next openslot, we skip slots, thereby more evenly distributing the items thathave caused collisions.
This will potentially reduce the clustering thatoccurs. Shows the items when collisionresolution is done with a “plus 3” probe.
This means that once acollision occurs, we will look at every third slot until we find onethat is empty. Figure 10: Collision Resolution Using “Plus 3”The general name for this process of looking for another slot after acollision is rehashing. With simple linear probing, the rehashfunction is (newhashvalue = rehash(oldhashvalue)) where(rehash(pos) = (pos + 1)% sizeoftable). The “plus 3” rehashcan be defined as (rehash(pos) = (pos+3)% sizeoftable). Ingeneral, (rehash(pos) = (pos + skip)% sizeoftable). It isimportant to note that the size of the “skip” must be such that all theslots in the table will eventually be visited. Otherwise, part of thetable will be unused.
To ensure this, it is often suggested that thetable size be a prime number. This is the reason we have been using 11in our examples.A variation of the linear probing idea is called quadratic probing.Instead of using a constant “skip” value, we use a rehash function thatincrements the hash value by 1, 3, 5, 7, 9, and so on. This means thatif the first hash value is h, the successive values are (h+1),(h+4), (h+9), (h+16), and so on. In other words,quadratic probing uses a skip consisting of successive perfect squares.shows our example values after they are placed usingthis technique.
Figure 11: Collision Resolution with Quadratic ProbingAn alternative method for handling the collision problem is to alloweach slot to hold a reference to a collection (or chain) of items.Chaining allows many items to exist at the same location in the hashtable. When collisions happen, the item is still placed in the properslot of the hash table. As more and more items hash to the samelocation, the difficulty of searching for the item in the collectionincreases.
Shows the items as they are added to a hashtable that uses chaining to resolve collisions. Q-2: Suppose you are given the following set of keys to insert into a hash table that holds exactly 11 values: 113, 117, 97, 100, 114, 108, 116, 105, 99 Which of the following best demonstrates the contents of the hash table after all the keys have been inserted using linear probing?. 100, , , 113, 114, 105, 116, 117, 97, 108, 99. It looks like you may have been doing modulo 2 arithmentic. You need to use the hash table size as the modulo value. 99, 100, , 113, 114, , 116, 117, 105, 97, 108.
Using modulo 11 arithmetic and linear probing gives these values. 100, 113, 117, 97, 14, 108, 116, 105, 99, ,. It looks like you are using modulo 10 arithmetic, use the table size. 117, 114, 108, 116, 105, 99, , , 97, 100, 113. Be careful to use modulo not integer division.
Implementing the Map Abstract Data TypeOne of the most useful Python collections is the dictionary. Recall thata dictionary is an associative data type where you can store key–datapairs.
The key is used to look up the associated data value. We oftenrefer to this idea as a map.The map abstract data type is defined as follows. The structure is anunordered collection of associations between a key and a data value. Thekeys in a map are all unique so that there is a one-to-one relationshipbetween a key and a value. The operations are given below.Map Create a new, empty map. It returns an empty mapcollection.put(key,val) Add a new key-value pair to the map.
If the key isalready in the map then replace the old value with the new value.get(key) Given a key, return the value stored in the map orNone otherwise.del Delete the key-value pair from the map using a statement ofthe form del mapkey.len Return the number of key-value pairs stored in the map.in Return True for a statement of the form key in map, ifthe given key is in the map, False otherwise.One of the great benefits of a dictionary is the fact that given a key,we can look up the associated data value very quickly. In order toprovide this fast look up capability, we need an implementation thatsupports an efficient search. We could use a list with sequential orbinary search but it would be even better to use a hash table asdescribed above since looking up an item in a hash table can approach(O(1)) performance.In we use two lists to create aHashTable class that implements the Map abstract data type. Onelist, called slots, will hold the key items and a parallel list,called data, will hold the data values. When we look up a key, thecorresponding position in the data list will hold the associated datavalue. We will treat the key list as a hash table using the ideaspresented earlier. Note that the initial size for the hash table hasbeen chosen to be 11.
Although this is arbitrary, it is important thatthe size be a prime number so that the collision resolution algorithmcan be as efficient as possible.Listing 2. Class HashTable: def init ( self ): self. Size = 11 self. Slots = None. self.
Data = None. self. Sizehashfunction implements the simple remainder method. The collisionresolution technique is linear probing with a “plus 1” rehash function.The put function (see ) assumes thatthere will eventually be an empty slot unless the key is already presentin the self.slots. It computes the original hash value and if thatslot is not empty, iterates the rehash function until an empty slotoccurs.
If a nonempty slot already contains the key, the old data valueis replaced with the new data value. Dealing with the situation where there areno empty slots left is an exercise.Listing 3. Def put ( self, key, data ): hashvalue = self. Hashfunction ( key, len ( self.
Slots )) if self. Slots hashvalue None: self. Slots hashvalue = key self. Data hashvalue = data else: if self. Slots hashvalue key: self.
Data hashvalue = data #replace else: nextslot = self. Rehash ( hashvalue, len ( self.
Slots )) while self. Slots nextslot != None and self. Slots nextslot != key: nextslot = self. Rehash ( nextslot, len ( self.
Slots )) if self. Slots nextslot None: self. Slots nextslot = key self.
Data nextslot = data else: self. Data nextslot = data #replace def hashfunction ( self, key, size ): return key% size def rehash ( self, oldhash, size ): return ( oldhash + 1 )% sizeLikewise, the get function (see )begins by computing the initial hash value.
If the value is not in theinitial slot, rehash is used to locate the next possible position.Notice that line 15 guarantees that the search will terminate bychecking to make sure that we have not returned to the initial slot. Ifthat happens, we have exhausted all possible slots and the item must notbe present.The final methods of the HashTable class provide additionaldictionary functionality. We overload the getitem andsetitem methods to allow access using````. This means thatonce a HashTable has been created, the familiar index operator willbe available.
We leave the remaining methods as exercises.Listing 4. Def get ( self, key ): startslot = self.
Hashfunction ( key, len ( self. Slots )) data = None stop = False found = False position = startslot while self. Slots position != None and not found and not stop: if self. Slots position key: found = True data = self. Data position else: position = self.
Rehash ( position, len ( self. Slots )) if position startslot: stop = True return data def getitem ( self, key ): return self.
Get ( key ) def setitem ( self, key, data ): self. Put ( key, data )The following session shows the HashTable class in action.
First wewill create a hash table and store some items with integer keys andstring data values.
Table of Contents
Summary
In this homework, you will implement a hash dictionary (also known as a hash map) and a hash set. We will be using these two data structures extensively in the next project.
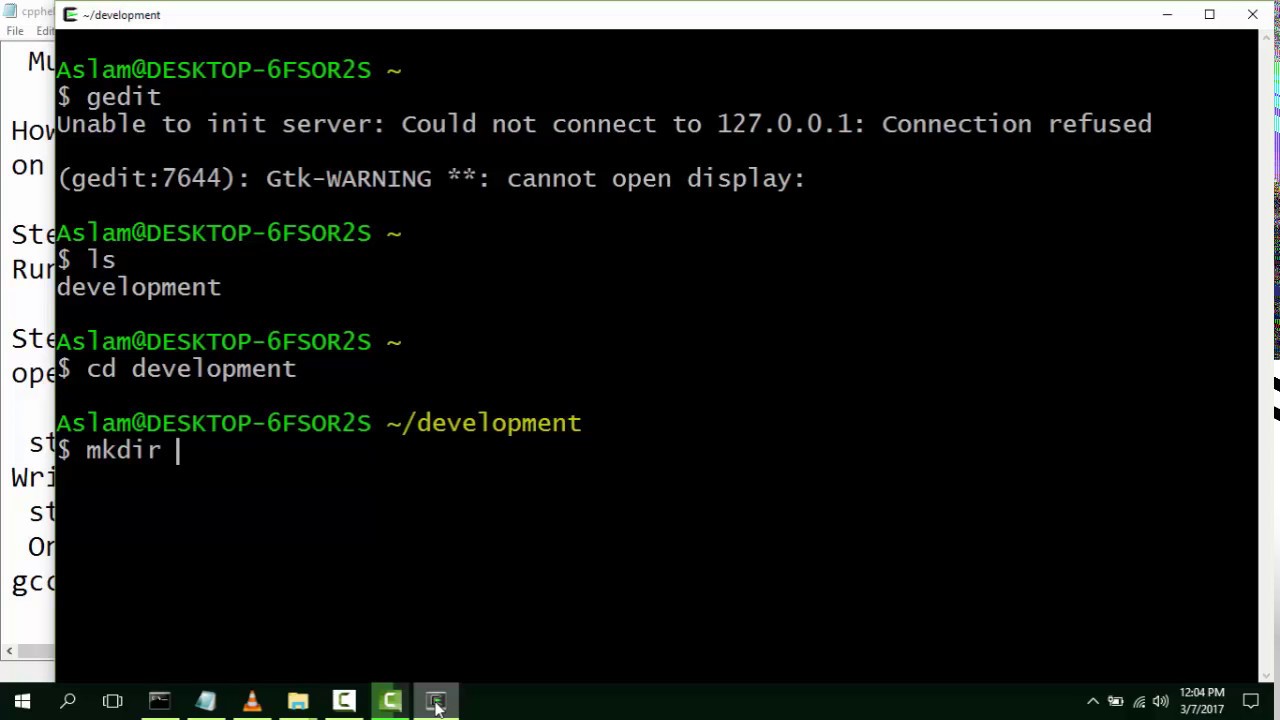
This entire project is due on Wednesday, July 31 at 11:59pm.
You will use these files from your prior assignments
src/main/java/datastructures/dictionaries/ArrayDictionary.java
src/main/java/datastructures/lists/DoubleLinkedList.java
If you have chosen a new partner for this assignment, choose either of your submissions from HW2 and verify that these are functioning properly.
You will be modifying the following files:
src/main/java/datastructures/dictionaries/ArrayDictionary.java
src/main/java/datastructures/dictionaries/ChainedHashDictionary.java
src/main/java/datastructures/sets/ChainedHashSet.java
Additionally, here are a few more files that you might want to review while completing the assignment (note that this is just a starting point, not necessarily an exhaustive list):
src/test/java/datastructures/dictionaries/BaseTestDictionary.java
src/test/java/datastructures/dictionaries/TestChainedHashDictionary
src/test/java/datastructures/sets/TestChainedHashSet.java
src/main/java/datastructures/dictionaries/IDictionary.java
src/main/java/datastructures/sets/ISet.java
src/main/java/analysis/experiments/*
Here's another video overview. Note: this video is from 19wi, so some info in this video may be a little outdated.
Expectations
Here are some baseline expectations we expect you to meet in all projects:
Follow the course collaboration policies
DO NOT use any classes from
java.util.*
. There are only two exceptions to this rule:You may import and use the following classes:
java.util.Iterator
java.util.NoSuchElementException
java.util.Objects
java.util.Arrays
You may import and use anything from
java.util.*
within your testing code.
DO NOT make modifications to instructor-provided code (unless told otherwise). If you need to temporarily change our code for debugging, make sure to change it back afterwards.
Section a: Set up project
Clone the starter code from GitLab and open the project in your IDE. See the instructions from Project 0 if you need a reminder on how to do this.
Copy your
DoubleLinkedList.java
andArrayDictionary.java
files from Project 1 to this new one.Copy your
DoubleLinkedList
delete
tests from Project 1 and paste them directly intoTestDoubleLinkedList.java
.Next make sure everything works.
Try running
SanityCheck.java
, and try running Checkstyle. Checkstyle should still report the same 5 errors withSanityCheck.java
as it did with Project 0.Try running
TestDoubleLinkedList
andTestArrayDictionary
, and make sure the tests still pass.
Section b: Implement new ArrayDictionary
constructor
In order to run one of the upcoming experiments, you will add an extra constructor to the existing ArrayDictionary
class. This constructor will be used when you implement ChainedHashDictionary
in the next part. The constructor should take in an integer representing the initial capacity of the pairs
array.
Below is the constructor stub you should implement:
Tip: to make sure this constructor is working, we can refactor our code to always use this new constructor. Try replacing your existing 0-argument constructor with the following code that will call your new constructor:
Afterwards, make sure the tests in TestArrayDictionary
still pass.
Section c: Implement ChainedHashDictionary
Task: Complete the ChainedHashDictionary
class.
In this task, you will implement a hash table that uses separate chaining as its collision resolution strategy.
Correctly implementing your iterator will be tricky—don't leave it to the last minute! Try to finish the other methods in ChainedHashDictionary
as soon as possible so you can move on to implementing iterator()
.
In the class when we covered separate chaining hash tables we used LinkedList
as the chaining data structure. In this task, instead of LinkedList
, you will use your ArrayDictionary
(from Project 1) as the chaining data structure.
When you first create your chains
array, it will contain null pointers. As key-value pairs are inserted in the table, you need to create the chains (ArrayDictionary
s) as required. Let's say you created an array of size 5 (you can create array of any size), and you inserted the key-value pair ('a', 11).
Your hash table should something like the following figure. In this example, the key 'a' lands in index 2, but if might be in a different index depending on your table size. Also, in this example, ArrayDictionary
(chain) is of size 3, but you can choose a different size for your ArrayDictionary
.
Now, suppose you inserted a few more keys:
Your internal hash table should now look like the figure below. In this example, keys 'a' and 'f' both hash to the same index (2).
Notes:
- The constructor you implement will take in a few parameters:
resizingLoadFactorThreshold
: if the ratio of items to buckets exceeds this, you should resizeinitialChainCount
: how many chains/buckets there are initiallychainInitialCapacity
: the initial capacity of eachArrayDictionary
inner chain - For the other, 0-argument constructor, you'll need to define some reasonable defaults in the final fields at the top of the class.
- Use
ArrayDictionary
for your internal chains/buckets.- Whenever you make a new
ArrayDictionary
, be sure to use your newArrayDictionary
constructor to correct set its initial capacity.
- Whenever you make a new
- If your
ChainedHashDictionary
receives a null key, use a hashcode of 0 for that key. - You may implement any resizing strategy covered in lecture—we recommend doubling the number of chains on every resize since it's the simplest to implement, though.
- We will be asking about your implementation design decisions later on, so it may be helpful to read ahead so you can keep this in mind while you implement
ChainedHashDictionary
. - Correctly implementing your iterator will be tricky—don't leave it to the last minute! Try to finish the other methods in
ChainedHashDictionary
as soon as possible so you can move on to implementingiterator()
. - Do not try to implement your own hash function. Use the hash method
hashCode()
that Java provides for all classes: so to get the hash of a key, usekeyHash = key.hashCode()
. This method returns an integer, which can be negative or greater thanchains.length
. How would you handle this? - Recall that operations on a hash table slow down as the load factor increases, so you need to resize (expand) your internal array. When resizing your
ArrayDictionary
, you just copied over item from the old array to the new one. Here, how would you move items from one hash table to another?
Notes on the ChainedHashDictionaryIterator
Restrictions, assumptions, etc.:
- You may not create any new data structures. Iterators are meant to be lightweight and so should not be copying the data contained in your dictionary to some other data structure.
- You may (and probably should) call the
.iterator()
method on eachIDictionary
inside yourchains
array, however, as instantiating an iterator from an existing data structure is both low cost in space and time. - You may and should add extra fields to keep track of your iteration state. You can add as many fields as you want. If it helps, our reference implementation uses three (including the one we gave you).
- Your iterator doesn't need to yield the pairs in any particular order.
- You should assume that a client will not modify your underlying data structure (the
ChainedHashDictionary
) while you iterate over it. For example, the following will never happen: Note that there are some tests that do something that looks similar but is different: they modify the dictionary in between creating new iterator objects, which is allowed behavior—it's okay to modify your data structure and then loop over it again, as long as you do not modify it while looping over it.
Tips for planning your implementation:
Before you write any code, try designing an algorithm using pencil and paper and run through a few examples by hand. This means you should draw the
chains
array that has some varying number ofArrayDictionary
objects scattered throughout, and you should try simulate what your algorithm does.Try to come up with some invariants for your code. These invariants must always be true after the constructor finishes, and must always be true both before and after you call any method in your class.
Having good invariants will greatly simplify the code you need to write, since they reduce the number of cases you need to consider while writing code. For example, if you decide that some field should never be null and write your code to ensure that it always gets updated to be non-null before the method terminates, you'll never need to do null checks for that field at the start of your methods.
As another example, it's possible to pose the
DoubleLinkedList
iterator's implementation in terms of invariants:- As long as the iterator has more values, the
next
field is always non-null and contains the next node to output. - When the iterator has no more values, the
next
field is null.
Additional notes:
- Once you've decided on some invariants, write them down in a comment somewhere so that you don't forget about them. We'll ask about these again in the writeup as well.
- You may also find it useful to write a helper method that checks your invariants and throws an exception if they're violated. You can then call this helper method at the start and end of each method if you're running into issues while debugging. (Be sure to disable this method once your iterator is fully working.)
- It may be helpful to revisit your main
ChainedHashDictionary
code and add additional invariants there to reduce the number of cases for thechains
array.
- As long as the iterator has more values, the
- We strongly recommend you spend some time designing your iterator before coding. Getting the invariants correct can be tricky, and running through your proposed algorithm using pencil and paper is a good way of helping you iron them out.
Section d: Implement ChainedHashSet
Task: Complete the ChainedHashSet
class.
In section c, you implemented the dictionary ADT with a hash table. You can also implement the set ADT using hash tables. Recall that sets store only a key, not a key-value pair. In sets, the primary operation of interest is contains(key)
, which returns whether a key is part of the set. Hash tables provide an efficient implementation for this operation.
Notes:
- To avoid code duplication, we will use an internal dictionary of type
ChainedHashDictionary<KeyType, Boolean>
to store items (keys) in yourChainedHashSet
. Since there are no key-values pairs (only keys) in sets, we will completely ignore the values in your dictionary: use a placeholder boolean whenever necessary. - Your code for this class should be very simple: your inner dictionary should be doing most of the work.
Section e (highly recommended): Consider more test cases
In this homework assignment, we won't be grading the tests that you write. But, to be thorough and to foster good habits, we strongly encourage you to write additional tests for your code, since they may help you spot different bugs or make you more confident in the correctness of your implementation. (Also remember that we have 'secret' tests that you will be graded on in addition to the provided tests, so it's in your best interest to test more cases.)
For this assignment, you should focus in particular on edge cases. Whenever you see conditional logic in your code (if statements, loop conditions, etc.) you should consider writing a test to check its edge cases. To make sure your tests are truly comprehensive, you should make sure that your test cases end up running every possible conditional branch in your code.
Although you can see the inner workings of your own code, it may sometimes be difficult to write code to check the actual state of your data structures by calling their public methods. Instead, you can test the state of your data structures by accessing your private fields directly; see the blue box below for more details.
Just like in Project 1, we've specified your fields to be package-private, which means the tests located in the same package can actually access the internal fields of your ChainedHashDictionary
and ChainedHashSet
.
The constructor tests in TestChainedHashDictionary.java
use a helper method to access the array of chains in your ChainedHashDictionary
; feel free to use the same helper method in your own tests.
Section f: Complete group write-up
Task: Complete a write-up containing answers to the following questions.
You and your partner will work together on this write-up: your completed write-up MUST be in PDF format. You will submit it to Gradescope. Log into Gradescope with your @uw.edu email address. When you submit, mark which pages correspond to the questions we ask, and afterwards, the partner who uploaded the PDF must add the other partner as a group member on Gradescope. Do not have each member submit individually. A video showing how to do this can be found here. We may deduct points if you do not correctly submit as a group or do not properly assign your pages/problems.
Design decisions
Before we get to the experiments, here are some questions about design decisions you made while doing the programming portion of the assignment.
ChainedHashDictionary
For this first prompt, reflect on a design decision you deliberately made while implementing your ChainedHashDictionary
. The specifications for this assignment are deliberately loose, so you should have needed to make some decisions on your own. Consider 1 such decision you had to make, and answer the following questions:
- What was the situation—what functionality were you implementing, and what details about it were left unspecified in the instructions?
- Describe at least two viable implementations that you considered.
- Describe the pros and cons of each solution you listed.
- What was your final choice? Why did you choose it over the other(s)—why did its pros and cons outweigh the pros and cons of the other(s)?
Your responses will be graded partially based on effort, and partially based on their clarity and how well they described your design decision. If you choose a design decision that had an obviously-best solution, or a problem in which you arbitrarily decided on a final solution, you may lose points.
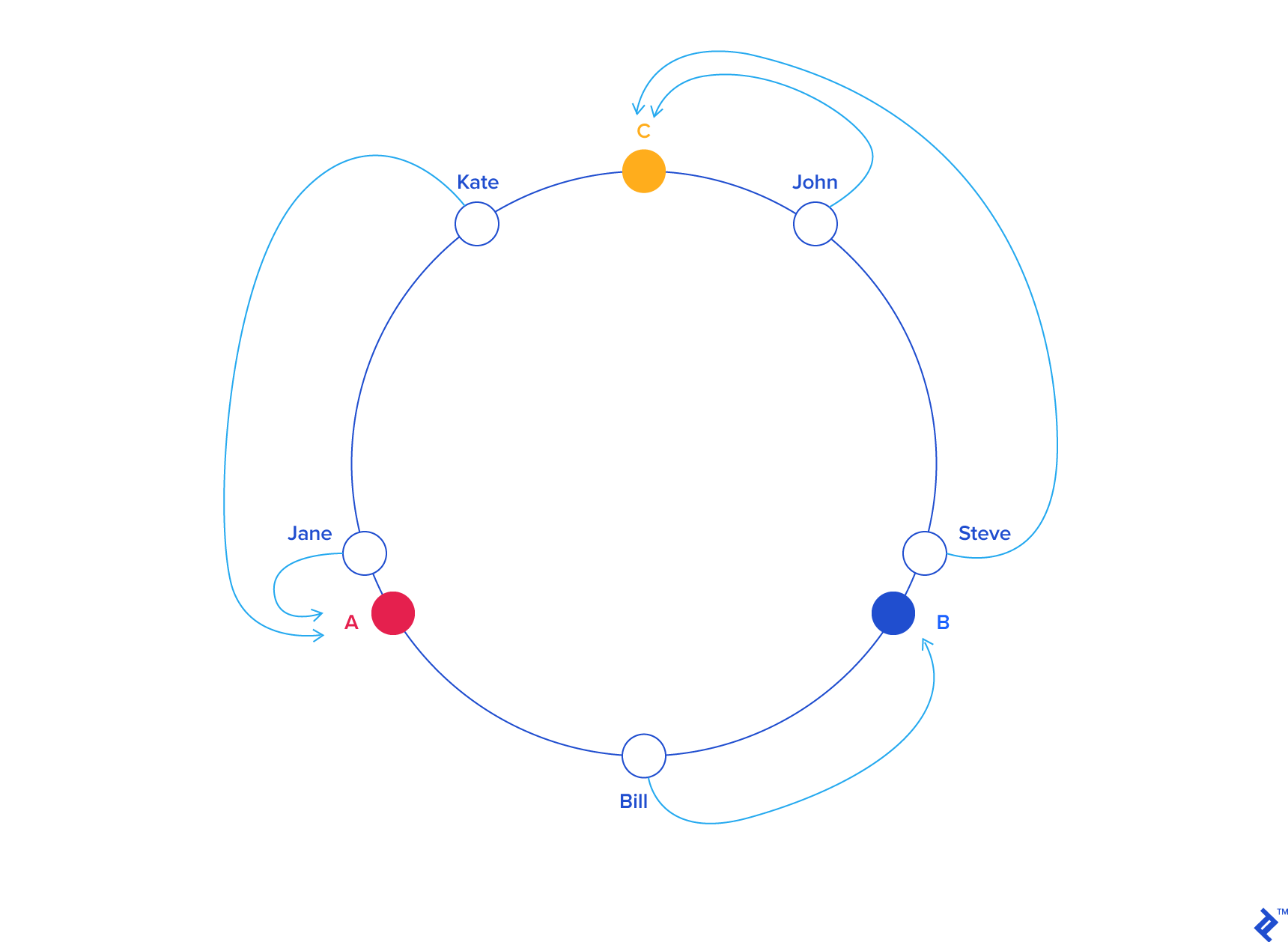
ChainedHashDictionaryIterator
For this prompt, briefly describe the invariant(s) you chose for your ChainedHashDictionary
iterator. Did you find them useful while implementing the iterator? Also note down any invariants you discarded for any reason (e.g., they were too inefficient/impossible to enforce, or they simply weren't useful).
This section will be graded based on completion.
Experiments
For each of the experiments, answer the bolded questions (and questions in the orange boxes) in your write-up. Just like before, a plot will automatically be generated to display the results of the experiments; include PNGs of the plots inside your write-up PDF.
The hypothesis/predict-based-on-the-code portions of your write-up will be graded based on completion, so just write down your honest thoughts before running the experiment. The post-experiment analysis portions will be graded based on the clarity of your explanations and whether they match up with your plot.
Experiment 1: Chaining with different hashCode
s vs. AVL trees
This experiment explores how different hash code functions affect the runtime of a ChainedHashDictionary
, and compare that to the runtime of an AVLTreeDictionary
.
First, we’ll look at the tests involving the ChainedHashDictionary
: test1
, test2
, and test3
. Each uses a different class (FakeString1
, FakeString2
, and FakeString3
respectively) as keys for a ChainedHashDictionary
. Each of the different fake string objects represent a string (by storing an array of chars) but each class has different implementations of the hashCode
method. Read over these implementations of hashCode
and take a look at the corresponding histograms (each plot shows the distributions of outputs of the hashCode
methods across 80,000 randomly-generated fake strings).
Below is a histogram for FakeString1
, the type of key used in test1
Below is the histogram for FakeString2
(test2
)
Dictionary
Below is the histogram for FakeString3
(test3
)
Now, predict which test method will have the fastest and slowest asymptotic runtime growths.
- Note that the distributions for the hash codes used in
test1
andtest2
look very similar in shape, but in your graphs you produce, you should see thattest2
(FakeString2
) tends to run much faster thantest1
(FakeString1
)—why is that? Hint: look at the x-axis scale labels. - You should also see that
FakeString3
produces the fastest runtimes when used as keys forChainedHashDictionary
—why is this? Explain using the histogram above, citing at least 1 observation about the histogram.
Now, we’ll consider test4
, which uses the AVLTreeDictionary
. This test uses a fake string class that does not provide a working hashCode
method, but is comparable in a way that mimics regular String
comparisons. You should see that the AVL-tree-based implementation performs much better than the chained-hash implementation when used with bad key objects, but looks like it’s only about a constant factor worse than chained-hashing with good keys.
- What functionality does
ChainedHashDictionary
require from its keys? What else must be true of its keys in order for the dictionary to perform well, if anything? - What functionality does
AVLTreeDictionary
require from its keys? What else must be true of its keys in order for the dictionary to perform well, if anything? - Which of these two has a runtime with a better (slower) asymptotic growth: the
AVLTreeDictionary
, or theChainedHashDictionary
(with good keys)? (Your answer should be based on the properties of these implementations, not just the results of this graph.)
Experiment 2: Load factor thresholds for resizing
This experiment tests the runtime for ChainedHashDictionary
's put
method across different values of the load factor threshold for resizing.
First, answer the following prompts:
- Briefly describe the difference between
test1
andtest2
. - Which test do you expect to run faster? What about asymptotically faster?
- Why is using the load factor of 300 slow? Explain at a high level how this affects the
ChainedHashDictionary.put
behavior. - This was not a part of this experiment, but explain why very small load factor thresholds (much less than 0.75; e.g., 0.05) might be wasteful.
Experiment 3: Initial internal chain capacities
This experiment tests the runtime for inserting elements into ChainedHashDictionary
with different initial capacities for the internal ArrayDictionary
chains.
Briefly describe the differences between the three tests.
Note that although the runtimes when using the three initial sizes are similar, using an initial capacity of 2 results in the fewest spikes and is generally the fastest. Why would a lower initial ArrayDictionary
capacity result in a more consistent and faster runtime?
Experiment 4: Data structure memory usage, take 2
C Program To Implement Dictionary Using Hashing Functions Example
This last experiment will estimate the amount of memory used by DoubleLinkedList
, ArrayDictionary
, and AVLTreeDictionary
as they grow in size. Predict the complexity class (constant, logarithmic, linear, (nlog(n)), quadratic, exponential) of memory usage for each of the 3 data structures the as its size increases.
Note: You may get the following warnings when the experiment; just ignore them:
WARNING: Unable to get Instrumentation. Dynamic Attach failed. You may add this JAR as -javaagent manually, or supply -Djdk.attach.allowAttachSelf
WARNING: Unable to attach Serviceability Agent. You can try again with escalated privileges. Two options: a) use -Djol.tryWithSudo=true to try with sudo; b) echo 0 | sudo tee /proc/sys/kernel/yama/ptrace_scope
- Describe the overall shapes of the graphs. Explain why two of them are similar but one is different.
- You should see that
test1
uses less memory thantest3
. Is the actual difference on your plot a difference in complexity classes or constant factors? What are some possible reasons that make the memory usages ofDoubleLinkedList
less thanAVLTreeDictionary
?
Section g: Complete individual feedback survey
Task: Submit a response to the feedback survey.
Write A C Program To Implement Functions Of Dictionary Using Hashing
After finishing the project, take a couple minutes to complete this individual feedback survey on Canvas. (Each partner needs to submit their own individual response.)
C Program To Implement Dictionary Using Hashing Functions Pdf
Deliverables
The following deliverables are due on Wednesday, July 31 at 11:59pm.
C Program To Implement Dictionary Using Hashing Functions Using
Before submitting, be sure to double-check that:
C Program To Implement Dictionary Using Hashing Functions
Submit by pushing your code to GitLab and submitting your writeup to Gradescope. If you intend to submit late, fill out this late submission form when you submit.